Blog
See all posts16/11/2022 - Journal Club
New to machine learning in proteomics? Check out the ‘ProteomicsML’ web platform
Machine learning approaches have become an established part of the mass spectrometry-based proteomics field in recent years. Several tools capable of predicting different aspects of peptide behavior have been developed and incorporated in data analysis workflows. These tools have proven to be beneficial in peptide and protein identification in proteomics experiments, and it is therefore of great interest to the people in the community to utilize these tools. It is of high importance to have datasets that are suitable for training and evaluation of machine learning models. However, it is often not a trivial task to prepare the data in a format that is compatible with the different software. At the moment there are different datasets available and often used for training on ProteomeTools, but there is not a formal consensus on which datasets to use for training and evaluation.
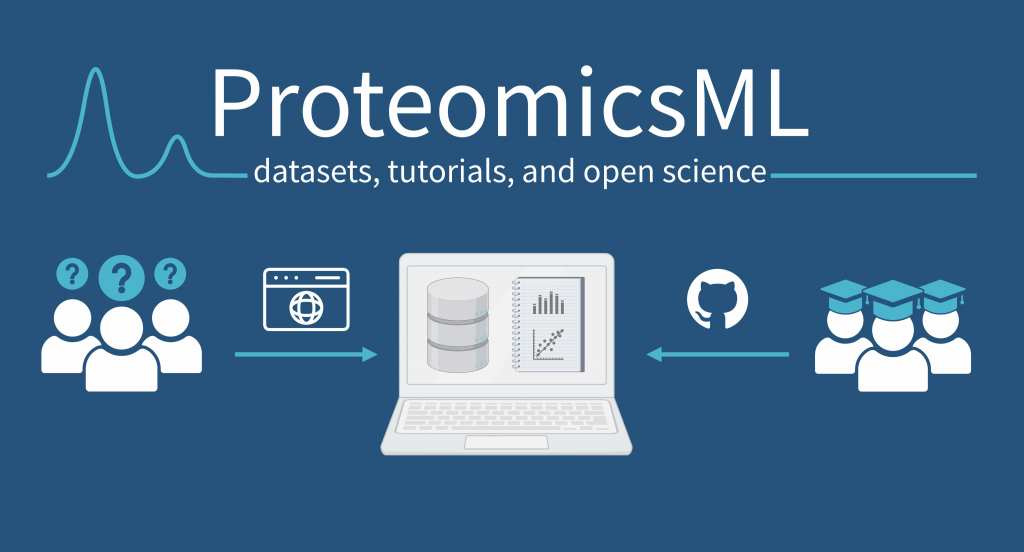
This is highlighted by Rehfeldt et al. in their paper “ProteomicsML: An Online Platform for Community-Curated Datasets and Tutorials for Machine Learning in Proteomics”, which is currently available as a preprint on ChemRxiv. As a result of the 2022 Lorentz Center Workshop on Proteomics and Machine learning (Neely et al. 2022 (submitted for review)), the authors have developed a web platform that they hope will bring together the people wanting to use machine learning tools in proteomics with the people developing them.
The authors have tried to overcome machine learning suitable training and evaluation barriers by providing access to datasets that are pre-processed and ready for applying machine learning models. On the ProteomicsML platform you can find tutorials on how to prepare your own data for the different state of the art machine learning models. Tutorials are available for four different data types representing different predictive capabilities in proteomics: retention time, fragment ion intensities, ion mobility and detectability. The platform also contains different datasets in the same four categories with varying complexity that you can download and explore on your own. If you have questions regarding any of the tutorials, you can ask a question in the Tutorials Q&A.
Besides providing tutorials and datasets, the authors also encourage people in the community to contribute themselves either by posting on one of the discussion boards or by contributing with their own dataset. If you want to contribute with datasets or tutorials there is also a very thorough guide that includes their code of conduct.
We think this is a great addition to the proteomics community as many of us PROTrEIN ESRs were newcomers to this field when we started our projects last year. A platform like this would have been a great starting point for us, and we hope that it will benefit many other people in the community! You can check out the ProteomicsML platform here https://proteomicsml.org/
Also, we could contact one of the authors of the paper, Ralf Gabriels, and interview him with the following questions:
Blog post team: In the paper you nicely describe why you think a platform like this would be useful to the proteomics community, but did you get direct feedback from people wanting to use the state-of-the-art machine learning tools or did you identify this challenge with different file formats and file complexity yourselves?
Ralf Gabriels: I think we mostly experienced these hurdles ourselves throughout our PhDs, and still do, of course. Dynamic and accessible educational resources are essential in a fast-growing, but complex field such as proteomics.
Blog post team: We know that the platform is still very new and in its start-up phase, but have you already had discussions about how/in which direction the content on the platform will grow?
Ralf Gabriels: Not too many discussions yet, but we do want to keep it up to date with developments in the field. Other than that, we are mostly looking towards the community and users to give feedback and feature requests on GitHub.
Blog post team: In which direction do you think that machine learning in proteomics will go over the next years?
Ralf Gabriels: I am certain that machine learning will continuously be more embedded in how we analyze the complex data that mass spectrometers produce. The better we understand how peptides behave in a mass spectrometer, the better we will be in interpreting the resulting data, and the better we will be at confidently identifying peptides (and thus proteins). Moreover, not only new instrumentation will drive the development of novel machine learning approaches, advancements in machine learning for proteomics will be able to optimize the development of the instruments, essentially opening a positive feedback loop between wet-lab and dry-lab methodological innovation.
Thanks a lot to Ralf Gabriels for his answers and for taking the time to answer our questions!
References
- Rehfeldt T, Gabriels R, Bouwmeester R, Gessulat S, Neely B, Palmblad M, et al. ProteomicsML: An Online Platform for Community-Curated Datasets and Tutorials for Machine Learning in Proteomics. ChemRxiv. Cambridge: Cambridge Open Engage; 2022; This content is a preprint and has not been peer-reviewed.
- Zolg DP, Wilhelm M, Schnatbaum K, Zerweck J, Knaute T, Delanghe B, Bailey DJ, Gessulat S, Ehrlich HC, Weininger M, Yu P, Schlegl J, Kramer K, Schmidt T, Kusebauch U, Deutsch EW, Aebersold R, Moritz RL, Wenschuh H, Moehring T, Aiche S, Huhmer A, Reimer U, Kuster B. Building ProteomeTools based on a complete synthetic human proteome. Nat Methods. 2017 Mar;14(3):259-262. doi: 10.1038/nmeth.4153. Epub 2017 Jan 30. PMID: 28135259; PMCID: PMC5868332.
Categories
Latest posts

08/05/2024 - Journal Club
Cross-Border Collaboration: Enhancing Peptide Identification with MS2Rescore and MS Amanda

08/09/2023 - Journal Club
Exploring Cellular Complexity: Unveiling Single-Cell Proteomics

23/08/2023 - Journal Club
Modeling Lower-Order Statistics to Enable Decoy-Free FDR Estimation in Proteomics